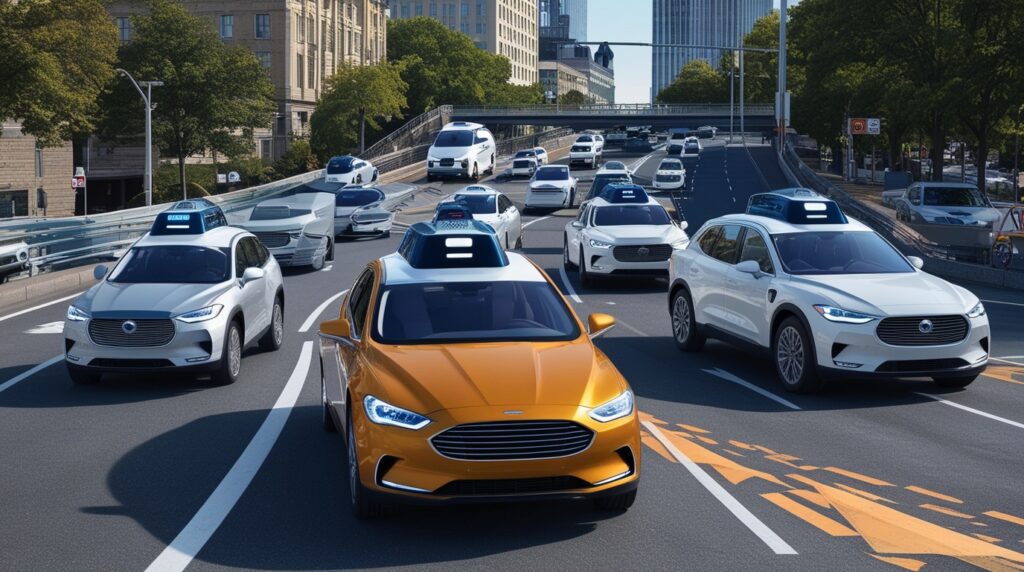
Introduction
The integration of autonomous vehicles (AVs) into real-life traffic situations marks a transformative shift in urban mobility and transportation management. As advancements in Artificial Intelligence (AI) and machine learning facilitate the rise of AVs, cities face both unprecedented opportunities and significant challenges. Autonomous vehicles, classified into levels of autonomy from Level 0 (no automation) to Level 5 (full automation), promise to enhance traffic flow by optimizing patterns, reducing congestion, and improving safety through real-time data processing and decision-making.
However, their incorporation into existing transportation systems necessitates substantial infrastructure changes and raises questions about regulatory frameworks, liability, and public trust in these technologies. Research indicates that the presence of even a small percentage of AVs can significantly improve traffic conditions by alleviating stop-and-go traffic phenomena commonly associated with human drivers. This potential for enhanced coordination and communication among vehicles may lead to smoother traffic movement, fewer accidents, and better fuel efficiency, thus contributing positively to urban sustainability.
Nonetheless, cities must address concerns related to congestion, environmental impacts, and the readiness of existing traffic management systems to accommodate AVs while ensuring equitable access for all transportation users. The dynamic and competitive AV industry, which includes major players like Waymo, Cruise, and Tesla, is rapidly evolving, with each company striving for advancements in autonomous technology integration.
As deployment increases, traffic patterns are expected to shift, prompting urban planners and policymakers to consider adaptive measures that foster safe and efficient mobility. Future scenarios envision mixed traffic environments comprising both human-driven and automated vehicles, which necessitate collaborative efforts to ensure effective adaptation of urban infrastructure and regulatory policies. Overall, the journey toward integrating autonomous vehicles into real-world traffic scenarios is multifaceted and complex.
It encompasses technological innovations, regulatory adaptations, and urban planning strategies aimed at optimizing traffic flow and enhancing overall quality of life in increasingly populated cities. As cities navigate this evolution, the collective approach of stakeholders will be crucial in addressing the unique challenges posed by autonomous driving technologies and ensuring a sustainable and efficient future for urban transportation systems.
Background
The integration of autonomous vehicles (AVs) into real-life traffic scenarios represents a significant evolution in transportation systems, driven by advancements in Artificial Intelligence (AI) and machine learning algorithms. AVs are categorized into different levels of autonomy, ranging from Level 0 (no automation) to Level 5 (full automation), with each level presenting unique challenges and opportunities for implementation in traffic flow management.
At lower levels of autonomy (L0-L2), driver assistance systems predominantly utilize rule-based and probabilistic methods for specific functions such as adaptive cruise control and lane departure warnings. As vehicles progress to higher levels of autonomy (L3-L4), machine learning and deep learning algorithms become essential, particularly for perception tasks like object detection and classification through convolutional neural networks (CNNs).
This shift enhances the ability of vehicles to navigate complex environments by interpreting real-time data from sensors such as LIDAR, radar, and GPS, thereby transforming traditional driving paradigms. The impact of AVs on traffic flow is multifaceted. They can potentially optimize traffic patterns by reducing congestion, improving safety, and enabling more efficient routing due to their capacity for real-time data processing and decision-making. Additionally,
AVs have the potential to facilitate increased mobility for non-drivers and individuals with disabilities, allowing greater independence in transportation options. As cities consider the incorporation of AVs, the necessity for comprehensive infrastructure adaptations becomes apparent. Cities will need to integrate advanced communication technologies and re-evaluate road designs to accommodate both traditional vehicles and AVs effectively.
The future of traffic management may increasingly rely on a blend of silicon technology and urban planning, which will be crucial in addressing the challenges posed by the rapid proliferation of autonomous driving technologies. The AV industry is dynamic and competitive, with numerous companies vying for market leadership in developing autonomous technologies.
These include notable names like Waymo, Cruise, and Tesla, each contributing to the ongoing evolution of autonomous driving capabilities and their integration into everyday traffic situations. As AV deployment begins to increase, it is expected that substantial shifts in traffic patterns and behaviors will accompany this transition, presenting both challenges and opportunities for urban mobility.
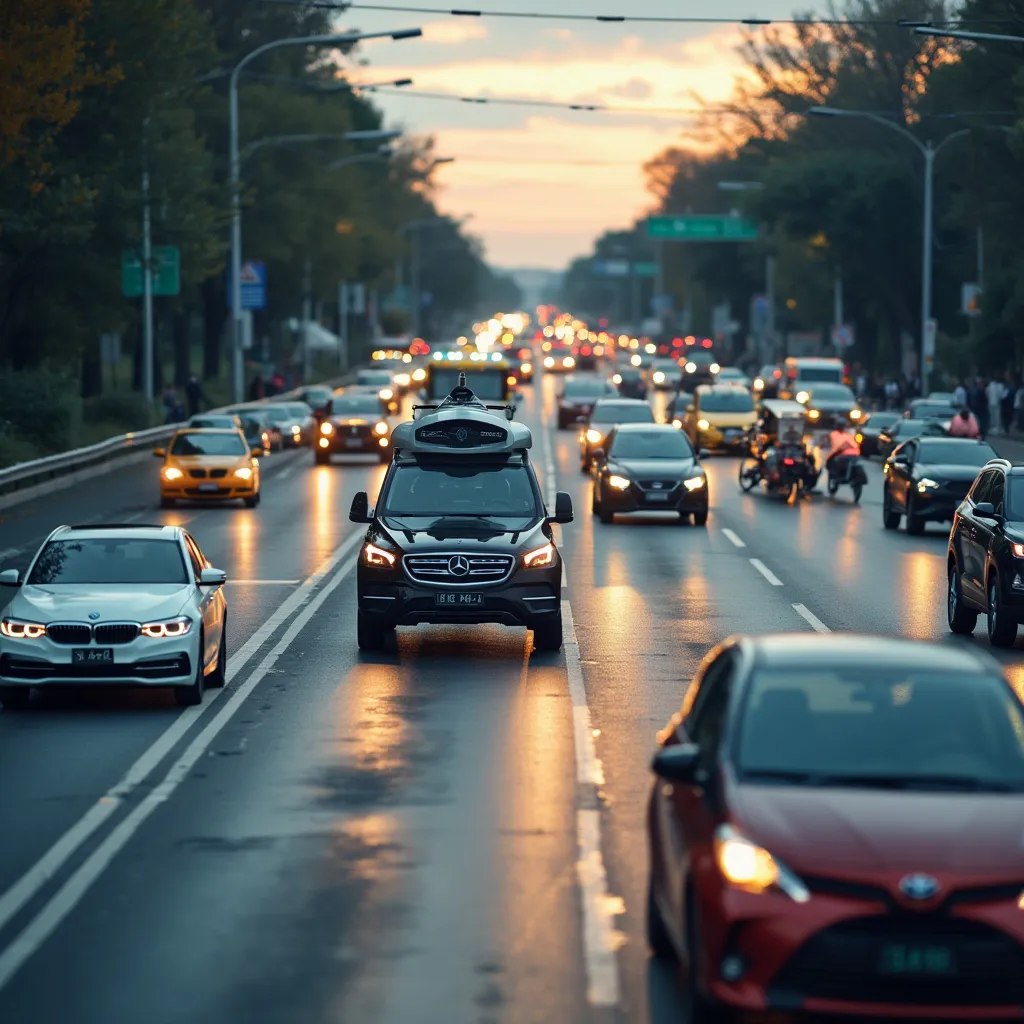
Traffic Flow Dynamics
Traffic flow dynamics in the context of autonomous vehicles (AVs) encompass various models and methodologies aimed at optimizing the movement of vehicles on road networks. This section discusses the application of machine learning (ML) and deep learning (DL) techniques, alongside traditional traffic flow models, to enhance traffic management and reduce congestion.
Machine Learning Applications
Machine learning techniques have been increasingly utilized to predict traffic flow and manage congestion effectively. Various models, including Convolutional Neural Networks (CNN) and Long Short-Term Memory (LSTM), have shown promising results in enhancing traffic prediction methodologies.
For example, studies have demonstrated that models like C-LSTM and STRCN outperform traditional baseline models such as ARIMA and Historical Average (HA) in terms of prediction accuracy and efficiency. These advancements contribute significantly to the development of intelligent transportation systems (ITS) in smart cities, aiming to provide seamless traffic management solutions.
Autonomous Vehicles and Traffic Efficiency
The integration of AVs into existing traffic systems has the potential to revolutionize traffic flow. Research indicates that even a small percentage of autonomous vehicles can significantly mitigate stop-and-go traffic phenomena caused by human drivers, leading to improved overall traffic conditions.
The operational capabilities of AVs allow for better coordination and communication, enabling smoother traffic movement and reduced accident risks. Studies have shown that controlled AVs can effectively eliminate phantom traffic jams, enhancing travel times and fuel efficiency for all vehicles on the road.
Simulation and Modeling
Traffic flow dynamics can also be analyzed through simulation models that incorporate both human-driven and autonomous vehicles. These simulations help researchers evaluate the effectiveness of different traffic management strategies and the impact of AV integration on overall traffic performance. For instance, the use of intelligent traffic lights controlled by deep reinforcement learning can optimize vehicle flow through intersections, reducing congestion and enhancing the efficiency of road usage.
Future Directions
As the field continues to evolve, future research should focus on experimental studies that further explore the application of advanced ML and DL techniques in traffic flow prediction. Integrating these methodologies with real-time data can help refine models and improve their predictive capabilities, ultimately contributing to smarter and more efficient traffic systems. Continued advancements in this area promise to enhance traffic flow dynamics and facilitate the seamless integration of autonomous vehicles into urban transportation networks.
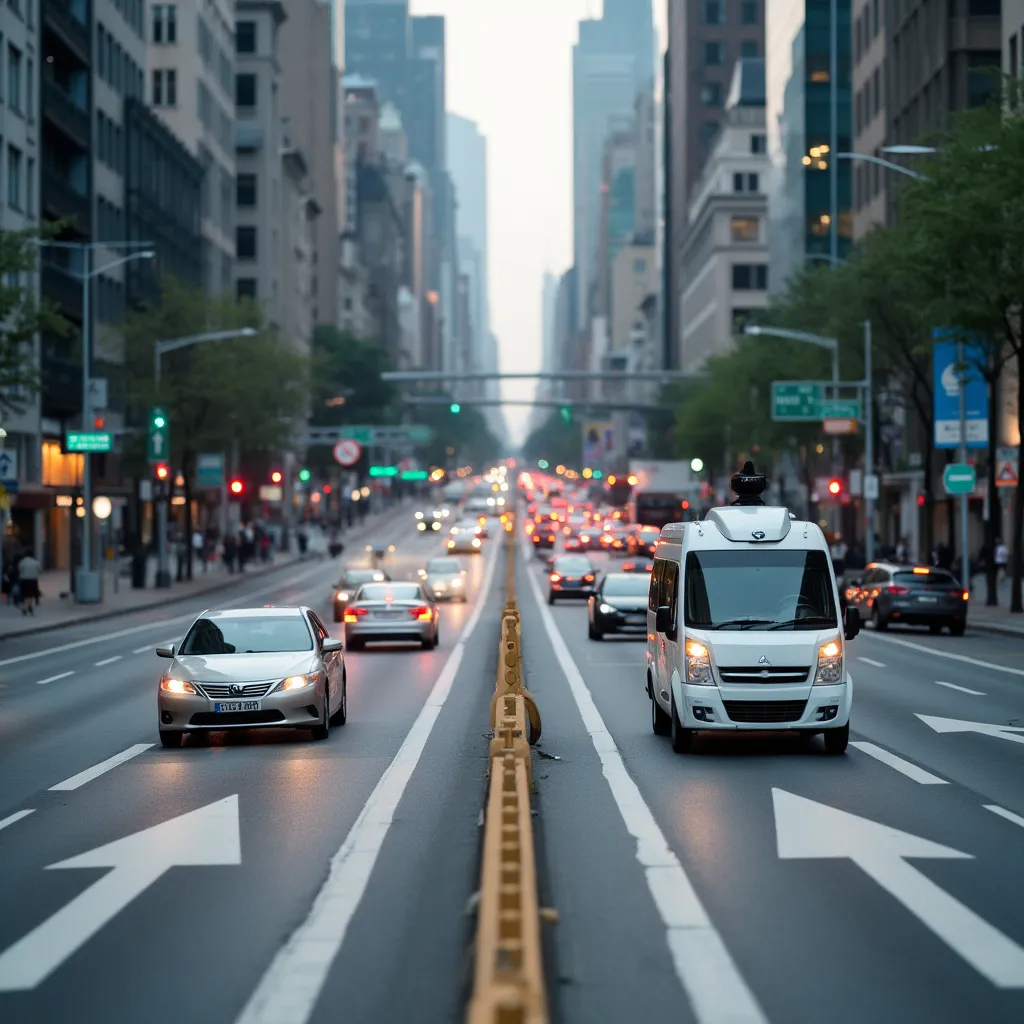
Real-life Traffic Situations
Transformative Advances in Traffic Management
The integration of data and innovative technologies in Intelligent Transportation Systems (ITS) represents a paradigm shift in urban mobility management. Cities are increasingly empowered to address modern transportation challenges through real-time data, facilitating informed decision-making. This evolution transitions urban mobility from a logistical task to a dynamic, intelligent adaptive system, ultimately enhancing citizen quality of life and fostering a more sustainable urban environment.
Simulation of Traffic Dynamics
Microscopic traffic simulations play a crucial role in understanding the interaction between conventional vehicles and automated vehicles (AVs) before their real-world deployment. These models are essential for evaluating the effects of automation on existing transportation systems, necessitating effective modeling techniques to accurately reflect the complex dynamics of driving behavior.
Various scenarios, such as high traffic demand, highlight how increased vehicle interactions can lead to deteriorated traffic conditions, stressing the importance of simulations in planning for the introduction of connected and automated vehicles (CAVs) in urban settings.
Challenges of Congestion and Urban Mobility
Traffic congestion remains a significant issue in urban environments, contributing to lost productivity and decreased quality of life. In 2018, congestion costs in the U.S. alone amounted to $87 billion, with megacities like Bogotá suffering the most, where drivers faced an average of 272 hours in traffic annually. Without proactive measures, traffic systems risk collapse, especially as urban populations grow. Projections indicate that by 2030, around 60% of the global population will reside in cities, intensifying existing issues related to emissions, congestion, space, and road accidents.
Strategic Planning for Future Mobility
In light of these challenges, city planners must adopt strategic measures to optimize urban mobility ecosystems. A key approach includes restricting private car usage to alleviate congestion. Municipal authorities are urged to envision their desired mobility future and implement policies that facilitate this vision. Stakeholders must address critical success factors and potential roadblocks to ensure the successful integration of AVs into existing traffic systems.
Real-World Implications of Automation
The introduction of autonomous vehicles into urban traffic presents both opportunities and challenges. As cities prepare for the arrival of self-driving vehicles, it is essential for all transportation stakeholders to learn from past experiences. Future scenarios suggest that mixed traffic environments, combining human-driven and automated vehicles, will require adaptive infrastructure and management practices to enhance safety and efficiency.
Furthermore, the successful integration of autonomous vehicles will depend on the collaborative efforts of city planners, industry experts, and policymakers to navigate the complexities of urban traffic dynamics.
Regulatory and Legal Framework
The regulatory landscape for autonomous vehicles (AVs) is complex and evolving, with a significant allocation of responsibilities between federal, state, and local authorities. In California, for example, the State Senate is actively addressing this issue; in May 2024, they passed SB 915 to restore some local control over the operation of self-driving cars while preventing local authorities from obstructing their safe operation. This illustrates the ongoing debate about how best to manage the integration of AVs within existing transportation frameworks.
Federal and State Regulation
Currently, the National Highway Traffic Safety Administration (NHTSA) holds the authority for vehicle safety but has not yet established comprehensive national safety standards for self-driving cars, despite its substantial engineering expertise. This gap creates regulatory uncertainty, particularly when computer software assumes the role of the driver. While NHTSA has issued guidance and a standing general order requiring the reporting of crash incidents involving AVs, definitive legal frameworks to address liability in accidents remain unclear. As of now, 29 states and Washington, D.C., have enacted legislation related to AVs, leading to a patchwork of regulations across the country.
Challenges and Mitigations
Several challenges exist within the current regulatory framework. One major issue is the lack of clear legal liability in cases of accidents involving autonomous vehicles. To address this, regulatory bodies are encouraged to develop frameworks that assign responsibility to manufacturers, software developers, and operators. Additionally, adapting existing traffic laws to better accommodate the unique capabilities and limitations of AVs is crucial. This could involve the establishment of new regulations that prioritize safety while considering ethical dilemmas inherent to automated decision-making.
Public trust in AVs is another critical factor that regulatory bodies must address. Many individuals express concerns regarding safety and the reliability of AI decision-making processes. To mitigate these fears, increasing transparency in testing procedures and demonstrating safety through rigorous validation will be essential. This will help build public confidence and pave the way for broader acceptance of autonomous driving technologies.
Future Considerations
The future of AV regulation will likely see a move toward more stringent testing and validation standards as well as international collaboration to harmonize regulations across borders. This will facilitate interoperability and safety in an increasingly interconnected transportation landscape. While various stakeholders, including state legislatures and federal agencies, continue to push for clearer guidelines, the path to establishing a cohesive regulatory framework remains a work in progress, requiring ongoing dialogue and adaptation to emerging technologies.
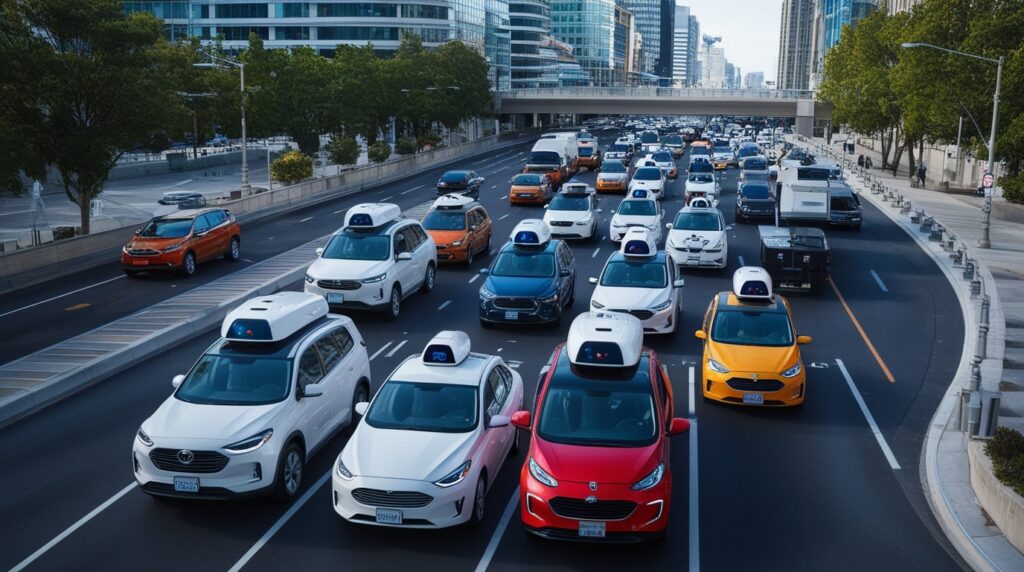
Environmental Impact
Autonomous vehicles (AVs) are poised to significantly influence environmental sustainability, particularly in urban settings. A major concern is their contribution to energy consumption and emissions. City-based vehicles account for 40% of all CO2 emissions from road transportation globally, with private cars being the primary culprits. AVs have the potential to minimize these impacts through various technologies, such as vehicle-to-grid integration and energy-efficient driving algorithms, which could optimize fuel consumption and reduce reliance on fossil fuels.
Energy Consumption and Emissions
The transition to AVs, especially electric and alternative fuel-powered vehicles, is expected to play a crucial role in reducing urban emissions. Research indicates that AVs could decrease energy consumption in ride-sharing scenarios by 0-20%. Furthermore, studies suggest that in optimistic scenarios, the total road transport energy could see a reduction of up to 40% with widespread AV adoption, while pessimistic views project increases in energy use due to other factors.
Eco-friendly Technologies
To ensure that AVs contribute positively to the environment, adopting eco-friendly technologies is vital. This includes minimizing the environmental impact of AVs during their lifecycle—production, operation, and disposal. Although AVs promise to enhance energy efficiency, they require substantial energy resources, making it critical to implement strategies that foster sustainability and reduce overall emissions.
Urban Planning and Public Health
The integration of AVs into urban environments requires careful planning to maximize their benefits while mitigating negative consequences. Cities must devise strategies to manage private car use, as unrestricted growth could lead to increased congestion and environmental degradation. AVs, particularly when used in shared mobility services, could alleviate urban traffic issues and lead to improvements in public health by reducing air pollution, which is linked to significant health risks and economic costs in urban areas.
Future Considerations
Looking forward, it is essential for municipal authorities to understand the implications of AV adoption and collaborate with manufacturers, technology firms, and other stakeholders. By planning ahead and investing in the necessary infrastructure, cities can position themselves to fully harness the potential environmental benefits of AVs, while addressing the challenges associated with their integration into existing transportation systems.
Integration with Existing Traffic Systems
Intelligent Transportation Systems (ITS)
Intelligent Transportation Systems (ITS) are pivotal in facilitating the integration of AVs with conventional vehicles, improving traffic flow and reducing congestion. These systems employ cooperative vehicle-to-vehicle (V2V) and vehicle-to-infrastructure (V2I) communication to enable real-time traffic coordination, optimize traffic signals, and support dynamic routing. As cities evolve towards smarter infrastructures, the application of machine learning (ML) in traffic flow prediction and management becomes increasingly vital. ITS aims to enhance mobility through creative solutions that address urban challenges, thereby making transportation safer and more efficient.
Traffic Assignment and Modeling
Traffic assignment involves determining the distribution of vehicles across a road network based on an Origin-Destination (O-D) matrix, which reflects the density of vehicles traveling between various points. Models such as Traffic Flow can be utilized to simulate these scenarios, where factors like vehicle count and road lengths are critical. For instance, simulations using 7,200 vehicles on road segments of 600 and 300 meters demonstrated the application of intelligent traffic lights controlled through deep reinforcement learning (RL) to improve overall traffic flow and mitigate congestion by rerouting vehicles to alternate paths.
Multi-Agent Reinforcement Learning Framework
Traditional traffic management systems often rely on single-agent RL methods or multi-agent algorithms focused solely on intersection delays. However, a new Multi-Agent RL framework is being proposed to enhance traffic management not only at intersections but also on surrounding roadways. By allowing agents to share experiences, the framework facilitates faster learning and improved performance. This coordinated rerouting of vehicles behind intersections to optimal routes significantly enhances traffic performance.
Integration of Autonomous Vehicles (AVs)
The integration of autonomous vehicles (AVs) into existing traffic systems presents both opportunities and challenges. Increasing the number of connected and automated vehicles (CAVs) can enhance traffic performance, especially when these vehicles can interact effectively with one another. As automation levels rise, especially beyond the yield point of 50-70%, the overall traffic dynamics improve, underscoring the need for advancements in technology to optimize traffic management systems.
Challenges in Traffic Control
Despite the advancements in traffic signal optimization, existing controllers often operate on fixed programs that do not adapt to real-time traffic conditions, which can lead to congestion. The complexity of traffic systems, characterized by nonlinear behaviors and large state-action spaces, complicates the development of effective control mechanisms. The necessity for sub-optimal control actions highlights the challenges faced in improving traffic system efficiency and mitigating congestion spread throughout the network.
Future Directions
The future of traffic management lies in the continued integration of smart technologies and advanced modeling approaches. By incorporating system utility metrics—such as traffic flow efficiency, average travel time, and fuel consumption—into existing control frameworks, traffic management can evolve into a multifaceted system that balances safety and efficiency. As innovations in IoT and machine learning further refine ITS capabilities, the potential for improved traffic management strategies in the context of mixed traffic scenarios will become increasingly attainable.